Ai Platform Pricing
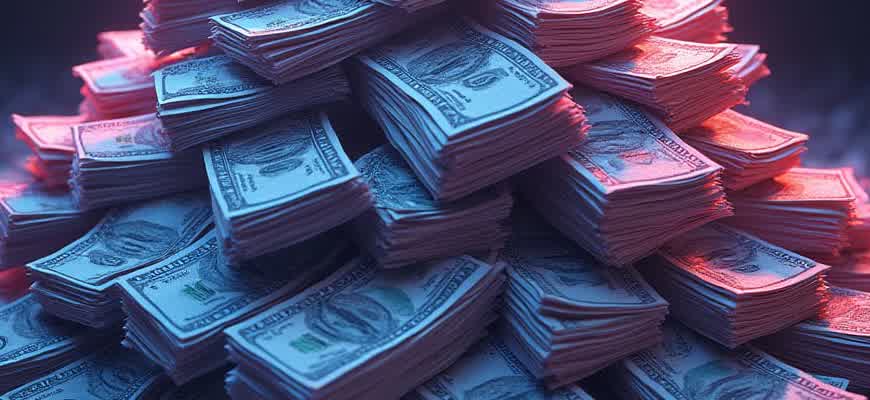
AI-driven platforms are becoming increasingly vital in the cryptocurrency industry, offering a variety of tools for trading, analytics, and market prediction. These platforms typically employ different pricing strategies depending on the features offered, usage levels, and user requirements. Below, we break down some common pricing structures for AI platforms used in crypto environments.
- Subscription-Based Pricing: Most AI platforms in the cryptocurrency space charge a monthly or annual fee, which gives users access to specific features, data sets, and AI models.
- Pay-As-You-Go: Some platforms follow a usage-based model, where users are billed according to their usage of computational resources or the number of API calls made.
- Freemium Model: A limited version of the platform is available for free, with premium features unlocked through paid plans.
Key Factors Influencing Pricing:
The pricing of AI platforms in the crypto market is largely determined by factors such as the number of transactions, computational power required, and the complexity of AI models integrated into the platform.
For users to make an informed decision, it's important to compare the different models. Below is a table highlighting some pricing options:
Platform | Pricing Model | Features |
---|---|---|
CryptoAI | Subscription | Real-time market analysis, automated trading |
TradeBotX | Pay-As-You-Go | Custom trading strategies, backtesting |
BotNet | Freemium | Basic trading signals, limited access to AI models |
AI Platform Pricing for Cryptocurrency Projects
When developing AI solutions tailored to the cryptocurrency market, pricing models for cloud-based AI platforms play a crucial role in determining your overall budget. Many platforms provide a variety of services that cater to machine learning, data processing, and predictive modeling, each with different pricing structures. These costs can vary greatly depending on the scale of your project, the volume of data processed, and the complexity of the algorithms involved.
Understanding these pricing tiers is key to selecting the right platform for your crypto-related initiatives. Whether you are building automated trading algorithms, implementing blockchain analytics, or optimizing transaction verification systems, knowing how pricing works can ensure that your investment in AI solutions aligns with your business objectives and budget constraints.
AI Platform Pricing Models in Cryptocurrency
Here are some common pricing models that AI platforms typically offer for cryptocurrency use cases:
- Pay-as-you-go: Charges are based on actual usage, such as the number of API calls, computational resources, or data storage.
- Subscription-based: Monthly or annual fees that grant access to a set amount of AI services.
- Tiered pricing: Different levels of service with distinct price points based on features and resource allocation.
Each model has its advantages depending on the nature and scale of your crypto project. For smaller startups or proof-of-concept solutions, pay-as-you-go might be more cost-effective, while larger organizations may benefit from a subscription or tiered pricing structure to control costs at scale.
Important: When working with large datasets such as transaction logs or blockchain history, consider the platform’s data storage and processing fees, as these can significantly increase the overall cost of the project.
Comparing AI Platforms for Cryptocurrency Solutions
Here’s a breakdown of some of the most popular AI platforms and their pricing for cryptocurrency-based applications:
Platform | Pricing Model | Average Cost |
---|---|---|
Google Cloud AI | Pay-as-you-go | From $0.10 per API call |
AWS AI | Subscription + pay-as-you-go | Starting at $0.25 per hour for basic instances |
Azure AI | Tiered Pricing | Starts at $5/month for basic services |
When selecting a platform, it's essential to account for both short-term costs (e.g., pilot projects) and long-term expenses (e.g., scaling operations). Make sure to evaluate both the technical requirements of your cryptocurrency use case and the pricing transparency of the platform to avoid unexpected costs.
Understanding the Core Components of AI Platform Pricing
When utilizing AI platforms in the cryptocurrency space, understanding the pricing structure is crucial for businesses and individual developers. These platforms typically charge for access to various machine learning and data processing tools, which are essential for tasks like blockchain analytics, cryptocurrency price forecasting, or fraud detection in decentralized finance (DeFi). By identifying the key elements that influence pricing, users can optimize costs while leveraging the full potential of AI technologies.
AI platforms generally break down their pricing into several core components, each with its own cost implications. These include computational resources, storage usage, data transfer fees, and specialized algorithms, all of which contribute to the total expense. Understanding how these components interact can help users make more informed decisions about which platform to choose and how to manage their usage effectively.
Core Elements Affecting AI Platform Pricing
- Compute Power: The most significant cost factor for AI platforms is often the computational resources required for running models. In cryptocurrency, AI models might need significant GPU or TPU power for processing large datasets from blockchain transactions or market data.
- Data Storage: The storage fees for large datasets, such as historical blockchain transactions or cryptocurrency exchange data, can add up quickly. These charges depend on the volume and duration of data stored.
- Data Transfer: Moving large datasets in and out of AI platforms can incur transfer fees. This is especially relevant when integrating external data sources like live market feeds or blockchain nodes.
- API Access: Many AI platforms offer APIs to access specific algorithms or tools for cryptocurrency analytics. These APIs are often priced based on usage, which can vary depending on the frequency and complexity of queries.
Cost Optimization Strategies
- Resource Scaling: Adjusting the scale of your AI model to match the computational demand can save costs. For instance, during off-peak hours, reducing the number of active processing units can lower expenses.
- Efficient Data Management: Minimizing the amount of data transferred and stored by applying data compression techniques or only keeping necessary historical records can help reduce storage and transfer costs.
- Long-term Contracts: Some AI platforms offer discounts for long-term contracts, so planning ahead and committing to extended usage can help lower overall costs.
Note: When evaluating pricing, it's important to consider hidden costs like API request limits or fees for using third-party services, which are common in AI platforms focused on cryptocurrency analytics.
Sample Pricing Structure
Service | Cost Model | Example |
---|---|---|
Compute Resources | Pay-as-you-go | $0.10 per compute hour |
Data Storage | Per GB stored | $0.02 per GB/month |
Data Transfer | Per GB transferred | $0.05 per GB |
API Access | Per 1000 requests | $0.05 per 1000 requests |
Key Factors Influencing AI Platform Costs for Businesses
In the rapidly evolving landscape of AI, the cost structure for businesses using AI platforms is not always straightforward. Several factors, such as computational power, data handling needs, and model complexity, significantly impact the pricing models provided by AI services. For businesses working with cryptocurrencies, these costs can further be influenced by the volatility of data and the specific requirements related to blockchain integration.
Understanding these costs is crucial for businesses to make informed decisions. The overall pricing is often determined by the platform's usage patterns, such as the volume of data processed, the frequency of model updates, and the level of support required. Businesses involved in crypto space must also consider factors like transaction speed, scalability, and security when selecting the right platform.
Factors Affecting AI Platform Pricing
- Data Storage and Processing Needs - High volumes of cryptocurrency transaction data demand efficient storage and real-time processing. AI platforms charge based on the amount of data processed and the complexity of queries.
- Compute Power and Scalability - The more computationally intensive the AI models (e.g., deep learning or predictive analytics for cryptocurrency trends), the higher the costs, especially with larger datasets.
- Custom Model Development - Tailored AI solutions that integrate blockchain technology or crypto-specific algorithms generally incur higher fees due to the bespoke nature of development.
Examples of Pricing Models
- Subscription-Based: Monthly fees depending on the tier of service (basic, professional, enterprise).
- Pay-As-You-Go: Costs are calculated based on actual resource usage (compute time, data processing).
- Enterprise Contracts: Custom pricing for large-scale, long-term commitments involving significant AI integration for financial analysis in cryptocurrency markets.
Note: Cryptocurrency-related AI platforms may also include additional charges for integrating with blockchain or securing data transactions, which can lead to unpredictable costs over time.
Factor | Impact on Pricing |
---|---|
Compute Power | Higher costs for models requiring more computational resources, especially for deep learning in real-time crypto trading applications. |
Data Complexity | Complex blockchain datasets or large-scale financial datasets increase storage and processing fees. |
Customization | Custom models tailored to specific business needs or blockchain integration add extra costs. |
Analyzing AI Pricing Models: Subscription vs Pay-Per-Use in the Cryptocurrency Sector
In the fast-evolving world of cryptocurrencies, AI platforms are increasingly becoming essential for data analysis, trading predictions, and portfolio management. As these technologies scale, the pricing models used to access them become critical to users, especially those working with dynamic cryptocurrency markets. Understanding the differences between subscription-based and pay-per-use pricing models is key to determining the most cost-effective approach for businesses and individual traders alike.
Subscription-based pricing provides users with a fixed cost over a specific time period, which can be advantageous for those who require consistent access to AI tools. On the other hand, the pay-per-use model charges users based on actual usage, making it more flexible and cost-efficient for businesses with variable or low-frequency needs. Both models have their advantages and disadvantages, which can impact profitability and scalability in the cryptocurrency space.
Key Differences between Subscription and Pay-Per-Use Models
- Predictability vs. Flexibility: Subscription offers predictable costs, while pay-per-use scales based on actual consumption.
- Cost Efficiency: Subscription can be more economical for high-volume usage, whereas pay-per-use benefits low or infrequent users.
- Access Frequency: Subscription often grants unlimited access during the subscription period, while pay-per-use limits users to the services used.
For crypto traders using AI tools, pay-per-use may be ideal for those looking to avoid large fixed costs in volatile market conditions.
Comparison Table: Subscription vs Pay-Per-Use
Aspect | Subscription-Based | Pay-Per-Use |
---|---|---|
Cost Structure | Fixed monthly or annual fees | Charges based on actual usage |
Scalability | Less flexible, may lead to overpaying | Highly scalable, pay only for what you use |
Access | Unlimited access during subscription period | Access limited to actual usage |
Predictability | Predictable costs, easy budgeting | Variable costs, harder to forecast |
For cryptocurrency businesses or traders with fluctuating AI needs, pay-per-use pricing offers significant savings without compromising access to essential tools.
How Volume of Usage Affects Pricing on AI Platforms
The pricing of AI platforms is highly dependent on the amount of resources consumed during usage. For cryptocurrencies, this dynamic is particularly relevant as decentralized applications (dApps) or blockchain-based services often need scalable computational power. The more transactions or computations a platform handles, the higher the costs associated with running its AI models, especially in high-frequency trading (HFT) or crypto mining algorithms.
Platforms typically adjust their pricing models based on usage volume, with varying tiers or rates applied to different levels of resource consumption. This is evident in the way blockchain-based AI systems charge for access to processing power, which can scale exponentially with network demands, influencing both startups and established projects in the cryptocurrency space.
Pricing Models for AI Platforms Based on Usage
- Pay-as-you-go pricing: Users pay for actual usage, ideal for low-frequency tasks or smaller crypto projects.
- Subscription-based pricing: A fixed monthly fee allows for predictable costs, often with varying tiers based on usage volume.
- Token-based pricing: Some platforms may require users to pay using specific tokens for access to resources, a common practice in decentralized AI systems built on blockchain.
As usage increases, platforms often introduce volume-based discounts to attract larger projects. Below is an example of how usage tiers might look based on AI resources required:
Usage Volume | Pricing Rate |
---|---|
Low (< 1000 requests) | Standard rate |
Medium (1000 - 5000 requests) | 5% discount |
High (> 5000 requests) | 15% discount |
Important: Increased usage can lead to network congestion, higher fees, or delayed processing times, especially in blockchain AI systems.
Managing Cryptocurrency Costs with AI Pricing Models
Cryptocurrency platforms often face significant operational challenges when it comes to controlling costs. The dynamic nature of the market means that usage and data demands fluctuate dramatically. One effective way to manage these costs is by implementing AI-driven pricing models that include tiered systems and custom packages tailored to individual needs. By aligning pricing with usage, cryptocurrency platforms can offer a more flexible solution that adapts to the demands of different users while keeping expenses predictable and manageable.
AI-powered pricing tiers and custom packages allow businesses to choose a structure that best fits their operational scale and resource requirements. This approach can lead to a more optimized allocation of computational power, which is critical for platforms that rely on continuous, high-volume transactions. The application of such pricing models also facilitates the use of machine learning to predict demand spikes, adjust prices dynamically, and avoid overpaying for unused resources.
Key Features of AI Pricing Models for Crypto Platforms
- Tiered Pricing: Pricing varies depending on the user's consumption level. Users with high transaction volumes can benefit from reduced rates.
- Custom Packages: These are tailored specifically to meet the unique needs of large investors or high-frequency traders, providing them with more cost-effective solutions.
- Usage-Based Pricing: Costs are directly tied to the amount of resources consumed, making the payment model fairer for both small and large users.
Example: Pricing Structure
Package Type | Transaction Volume | Price |
---|---|---|
Basic | Up to 1,000 transactions/month | $50/month |
Pro | 1,001 - 10,000 transactions/month | $200/month |
Enterprise | 10,001+ transactions/month | Contact for Pricing |
"With AI-based pricing strategies, cryptocurrency platforms can not only optimize their operational costs but also ensure that they remain competitive in an ever-evolving market."
Hidden Costs in AI Platforms: What You Need to Know
When engaging with AI platforms, there are often hidden financial challenges that businesses fail to account for, especially when they integrate cryptocurrency-based solutions. These costs can easily accumulate, leading to unexpected expenses. For cryptocurrency projects relying on AI for blockchain analysis, smart contract audits, or automated trading, understanding the total cost structure becomes crucial. Let's explore the less obvious costs you might encounter when using these advanced platforms.
Although AI platforms often advertise low base prices, several additional fees can inflate the final bill. These include transaction fees, data storage costs, and charges for using AI model training on high-performance infrastructure. In the context of blockchain technologies, these hidden fees can significantly impact overall profitability, especially with volatile crypto market conditions. Let’s break down some common hidden costs to watch for.
Common Hidden Expenses in AI Platforms
- Transaction Fees: These are often overlooked but can add up quickly when executing smart contract operations or blockchain transactions on AI-powered platforms. Blockchain networks usually charge per transaction, and costs can vary depending on network congestion.
- Data Storage: AI platforms often require large datasets for training models. Storing these datasets in decentralized storage systems can incur substantial costs, especially when dealing with high-volume blockchain transactions or logs.
- Computational Costs: Running AI models for tasks like real-time data processing or automated trading may require high computational resources. If you’re utilizing cloud-based or decentralized AI platforms, these costs could be tied to usage times, often billed hourly.
Examples of Additional Charges
- Cloud Computing Resources: Many AI platforms provide cloud infrastructure but charge extra for GPU or specialized hardware required for processing large-scale cryptocurrency data.
- API Calls and Integrations: Certain AI services offer APIs to interact with blockchain networks or crypto data, but frequent API calls may lead to incremental charges.
- Model Customization: Customizing AI algorithms or integrating specialized machine learning models may involve consultation fees or premium features.
Important: Always review the pricing tiers of AI platforms, especially when working with cryptocurrencies. Some hidden costs, such as network-specific transaction fees, may not be immediately apparent in the initial quote but can significantly affect your bottom line.
Cost Breakdown Comparison
Cost Type | Blockchain Application | Standard AI Model |
---|---|---|
Transaction Fees | High (based on network activity) | Low to Medium |
Data Storage | Varies (Decentralized storage) | Medium (cloud storage) |
Computational Costs | High (due to data volume) | Medium (GPU usage) |
Estimating and Forecasting AI Platform Costs for Cryptocurrency Projects
As cryptocurrency platforms leverage artificial intelligence (AI) to enhance trading strategies, blockchain security, and market predictions, it is crucial to manage costs effectively. Estimating expenses for AI tools requires a strategic approach, considering both fixed and variable charges associated with computing power, data storage, and processing. Understanding these elements will help determine accurate forecasts and budget allocations.
AI infrastructure costs in the cryptocurrency sector can fluctuate based on platform usage, algorithm complexity, and integration with blockchain systems. By focusing on resource consumption, cryptocurrency projects can assess costs with precision and avoid unexpected financial burdens. Below are some key factors that influence pricing and strategies to forecast AI platform expenses.
Key Cost Factors
- Compute Resources – The cost of processing power for machine learning models, especially for large-scale blockchain data analysis, can vary significantly.
- Storage – Cryptocurrency data is vast and grows rapidly, demanding scalable storage solutions.
- Data Access Fees – Access to real-time data streams, especially market data, may incur additional charges.
Forecasting AI Platform Costs for Cryptocurrency
- Analyze Historical Data – Reviewing past usage patterns can provide insights into future consumption, especially for mining pools or decentralized finance (DeFi) applications.
- Project Future Growth – Consider the potential increase in transactions or smart contract executions as the crypto platform expands.
- Utilize AI Cost Calculators – Many AI providers offer online tools to help forecast charges based on specific requirements.
Accurate forecasting of AI platform costs ensures the scalability and efficiency of cryptocurrency operations while minimizing the risk of exceeding budget limits.
Example Cost Breakdown
Service | Cost Type | Estimate (Monthly) |
---|---|---|
Compute Power | Fixed | $3,000 |
Storage (Data Blocks) | Variable | $1,500 |
Data Access | Variable | $2,000 |
The Impact of AI Platform Pricing on ROI for Small to Medium Enterprises
As small to medium enterprises (SMEs) increasingly adopt AI platforms, the cost of using these technologies plays a crucial role in determining their return on investment (ROI). The pricing structures of AI platforms can vary widely, influencing the affordability and scalability of AI integration for businesses operating on tighter budgets. In particular, the cryptocurrency sector, where volatility and market fluctuations are common, faces unique challenges when it comes to integrating AI solutions. The proper pricing model can either enable SMEs to leverage AI for competitive advantage or push them into unmanageable expenses.
For SMEs within the crypto space, which often deal with high transaction volumes and require real-time data analysis, the decision to integrate AI must balance the initial costs with long-term returns. Several factors influence the ROI from AI investments, and understanding platform pricing is critical to ensuring that businesses can achieve sustainable profits without overextending their financial capabilities. The following points illustrate how pricing models directly impact ROI for crypto-based SMEs:
Key Factors Influencing ROI for SMEs in Crypto
- Pricing Flexibility: A tiered pricing model allows SMEs to scale their AI usage according to their growth, enabling them to manage costs effectively.
- Performance Efficiency: Platforms offering pay-as-you-go pricing based on usage ensure that SMEs only pay for the resources they actually need, thus maximizing their return on investment.
- Support and Integration: Platforms with lower upfront costs but strong integration support and guidance can reduce operational overhead and improve the overall ROI.
AI platforms that offer flexible, usage-based pricing allow SMEs in the crypto sector to scale their AI investments without excessive upfront costs, making them ideal for businesses with fluctuating needs.
AI Pricing Models: Pros and Cons
Pricing Model | Pros | Cons |
---|---|---|
Subscription-Based |
|
|
Pay-As-You-Go |
|
|
Freemium |
|
|