5 Best Ai Stocks
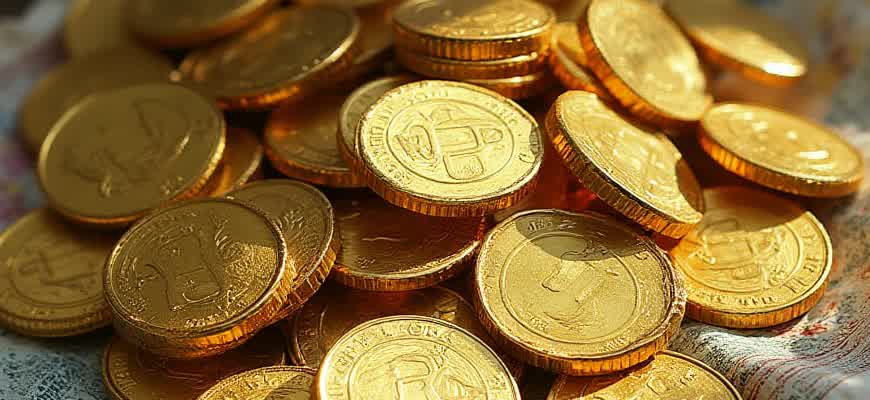
Artificial intelligence is reshaping the blockchain ecosystem, and several publicly traded companies are leading the charge by integrating AI into decentralized technologies. These firms aren't just dabbling–they’re deploying AI to optimize crypto mining, detect fraud, and power autonomous financial systems.
Below is a breakdown of five influential corporations utilizing machine learning and neural networks to gain a competitive edge in the crypto sector:
- NVIDIA Corporation
- Alphabet Inc. (Google)
- Microsoft Corporation
- IBM
- Palantir Technologies
Insight: Companies at the intersection of AI and crypto are not only enhancing blockchain infrastructure but also fueling the next wave of decentralized finance.
Company | AI Application | Crypto Relevance |
---|---|---|
NVIDIA | GPU acceleration for deep learning | Blockchain analytics and mining optimization |
Alphabet | AI modeling via Google Cloud AI | Smart contract auditing, DeFi infrastructure |
Microsoft | Azure AI for blockchain platforms | Enterprise-grade decentralized apps |
IBM | Watson for AI-driven smart contracts | Supply chain transparency using blockchain |
Palantir | Data integration with AI forecasting | Predictive modeling for crypto markets |
Top AI-Driven Tech Equities with Crypto Integration Potential in 2025
Artificial intelligence is reshaping not only traditional markets but also the landscape of decentralized finance. A number of public tech entities are heavily investing in neural networks, data processing, and blockchain interoperability–key elements where AI and crypto intersect. These developments position certain companies as strategic assets for investors who are bullish on both sectors.
In 2025, AI is expected to enhance crypto trading automation, blockchain security, and on-chain analytics. Forward-looking investors are closely watching firms that not only lead in AI development but also explore applications in digital currencies and decentralized infrastructures.
Public Tech Entities Poised for AI-Crypto Synergy
Strong integration of AI with blockchain offers unmatched predictive capabilities and system efficiency in crypto environments.
- NVIDIA Corp. – Supplies GPUs essential for AI model training and crypto mining.
- Alphabet Inc. – DeepMind’s algorithms have implications for smart contract optimization.
- Meta Platforms – Invests in AI and metaverse, with potential for Web3 convergence.
- Microsoft Corp. – Azure AI services already cater to blockchain startups.
- Palantir Technologies – Known for big data and predictive analytics, applicable to DeFi trends.
- Look for companies with both AI patents and blockchain partnerships.
- Evaluate R&D spending toward decentralized tech integration.
- Monitor strategic acquisitions in the AI-crypto ecosystem.
Company | AI Focus | Crypto Relevance |
---|---|---|
NVIDIA | Hardware for model training | Mining and neural inference |
Alphabet | AI research (DeepMind) | Smart contract logic models |
Microsoft | Cloud AI platforms | Blockchain integrations |
How to Spot Promising AI Investments in the Crypto Market
As artificial intelligence technologies continue to reshape the digital landscape, crypto investors are increasingly turning their attention to blockchain projects integrating advanced machine learning and data processing models. These integrations aren't just experimental–they're becoming essential components for scalability, security, and predictive analytics in decentralized ecosystems.
To assess which crypto-based AI initiatives may offer long-term returns, it's important to look beyond hype and focus on metrics like tokenomics, data utility, and real-world use cases. The convergence of AI and blockchain often materializes in sectors such as DeFi optimization, fraud detection, and autonomous trading algorithms.
Evaluation Criteria for Long-Term Growth
Important: A crypto project’s AI model must demonstrate verifiable value within decentralized infrastructure–not just promise future potential.
- On-chain AI Integration: Analyze how AI is embedded into smart contracts or used to automate core blockchain functions.
- Developer Activity: Review GitHub repositories and community contributions to ensure ongoing innovation and security updates.
- Token Utility: Check if the native token is essential for accessing AI services, not just speculative trading.
- Start by evaluating market capitalization relative to project maturity.
- Study partnerships with data providers, cloud AI platforms, or research institutions.
- Assess scalability and whether the AI model improves transaction throughput or reduces gas fees.
Project | AI Use Case | Growth Indicator |
---|---|---|
Fetch.ai | Autonomous agent-based systems | Increasing real-world integrations |
Ocean Protocol | Decentralized data marketplaces | Rising data token adoption |
Numerai | AI-powered hedge fund signals | Consistent model improvements |
Key Financial Indicators for Assessing Blockchain-Driven AI Enterprises
Blockchain-integrated AI firms are drawing intense investor attention due to their scalability and decentralized infrastructure. To make informed investment decisions, it's essential to evaluate not only their technical innovations but also a set of core financial indicators that reveal real-world viability and long-term profitability.
These companies often operate in volatile environments, especially when tokens or on-chain governance are involved. Therefore, financial due diligence requires a focus on metrics that reflect operational efficiency, revenue growth, and tokenomics alignment with shareholder interests.
Essential Metrics to Analyze
- Recurring Revenue: Prioritize firms generating consistent, subscription-based income through AI-as-a-service (AIaaS) models on decentralized platforms.
- Gross Margin: High margins indicate efficient AI infrastructure utilization and optimized blockchain resource costs.
- Token Circulation vs. Revenue: Assess the balance between token supply growth and actual platform revenue to avoid inflation-driven dilution.
For tokenized AI firms, an increasing revenue-to-circulating-token ratio often signals stronger utility and demand for the native asset.
Metric | Why It Matters |
---|---|
Cash Burn Rate | Shows how fast the company spends capital; critical for early-stage blockchain AI ventures. |
Partnership Revenue | Measures success in integrating AI protocols into external blockchain ecosystems. |
Node Incentive Cost | Reflects the expense of maintaining decentralized compute infrastructure. |
- Compare annualized returns with traditional AI firms to benchmark blockchain integration benefits.
- Evaluate governance token utility–whether it contributes to platform growth or merely speculative activity.
A decentralized AI firm with low burn rate and high node engagement cost-effectiveness is typically positioned for long-term sustainability.
AI-Driven Income Models in the Crypto Sector: A Comparative Overview
The integration of AI in blockchain ecosystems creates new pathways for sustainable income. Startups and DAOs are adopting enterprise-level AI solutions, packaged as software services or algorithm access points. The monetization strategy largely determines scalability and investor interest.
Revenue Mechanisms in AI-Infused Crypto Projects
- Subscription Platforms (SaaS): Tokenized access to AI tools via recurring payments. Often implemented with tiered smart contracts.
- Algorithm Licensing: Renting proprietary trading or predictive models to third-party platforms or protocols.
- Decentralized Cloud Solutions: Offering GPU compute power or AI model hosting through distributed infrastructure (e.g., Akash, Golem).
Strong monetization models not only attract capital but also reduce dependency on speculative token value.
Model | Revenue Stability | Scalability | Use Case Example |
---|---|---|---|
Token-Gated SaaS | Medium | High | AI copywriting tools for NFT marketing |
IP Licensing | High | Medium | Predictive models for DeFi risk scoring |
Distributed AI Cloud | Variable | High | AI training-as-a-service using idle crypto nodes |
- Tokenomics should support long-term utility, not just speculative demand.
- Decentralization of compute must align with AI performance requirements.
- Licensing frameworks need clarity on IP ownership and revenue sharing.
Analyzing R&D Spending as a Signal of Blockchain-Driven AI Advancements
Firms with significant R&D allocations are frequently those developing on-chain machine learning infrastructure, optimizing smart contract automation, or deploying neural networks for real-time fraud detection. Analyzing these expenditures offers insight into which crypto-aligned enterprises are not only participating in the AI race, but shaping its frontier technologies.
Key Indicators of Tech-Forward Crypto Projects
Companies with over 15% of annual revenue allocated to R&D are statistically more likely to produce patentable AI-blockchain solutions within 18 months.
- Protocol Enhancement: Projects like Fetch.ai and SingularityNET reinvest heavily in training AI models on decentralized data sets.
- Security Automation: Startups focused on zero-knowledge proofs and anomaly detection use AI to secure DeFi layers.
- Infrastructure Scaling: Teams are channeling R&D into AI-powered load balancing across Layer-2 chains.
Project | R&D Spend (% of Revenue) | AI Use Case |
---|---|---|
SingularityNET | 28% | Decentralized AI marketplaces |
Ocean Protocol | 22% | AI data monetization frameworks |
Numerai | 31% | Meta-model training on encrypted data |
- Monitor quarterly R&D reports from AI-token ecosystems.
- Evaluate partnerships with academic and AI research labs.
- Track on-chain deployments of newly funded AI features.
Understanding Market Positioning in the AI Ecosystem
In the rapidly evolving landscape of artificial intelligence, blockchain-based infrastructure is emerging as a critical player. Decentralized AI platforms are leveraging smart contracts to provide secure, scalable, and transparent environments for data processing and algorithm execution. This shift enables crypto-native projects to position themselves as foundational layers for AI deployment, challenging traditional cloud-based incumbents.
Projects integrating both AI and decentralized finance (DeFi) are gaining traction due to their ability to autonomously allocate capital, optimize liquidity across protocols, and automate yield generation. These innovations are creating a new class of digital assets that are inherently intelligent and capable of adapting to market conditions without human intervention.
Key Differentiators for Blockchain-AI Projects
- Data Sovereignty: Users retain ownership of their data while contributing to model training via tokenized incentives.
- On-chain Compute: Projects like Fetch.ai and Bittensor offer distributed networks for AI model inference and training.
- Token Economics: Native tokens govern model access, staking, and value exchange across AI services.
Decentralized AI protocols challenge centralized monopolies by democratizing access to computational intelligence and incentivizing global participation.
Project | Core Focus | Token Utility |
---|---|---|
Ocean Protocol | Data Marketplace for AI | Staking, Governance, Access Control |
SingularityNET | AI-as-a-Service | Payment for AI Services, DAO Voting |
Bittensor | Decentralized Neural Networks | Model Validation, Reward Distribution |
- Evaluate token demand in relation to AI utility.
- Monitor partnerships with real-world data providers.
- Track protocol adoption via on-chain activity metrics.
How to Evaluate AI Integration in a Company's Product Development Strategy
Assessing how a company integrates artificial intelligence (AI) into its product development pipeline is crucial for understanding its potential for growth in the tech and crypto sectors. AI technology can significantly enhance product innovation, increase efficiency, and provide a competitive edge. For companies working in cryptocurrency or blockchain, AI adoption can streamline complex processes, from predictive analytics for market trends to automation in smart contracts and fraud detection systems.
To evaluate AI integration effectively, it is important to understand how AI fits into the broader business model, its impact on existing products, and how it influences future innovations. Companies must demonstrate clear objectives, such as enhancing user experiences, reducing costs, or providing more secure solutions in the cryptocurrency space. Understanding the extent to which AI contributes to achieving these objectives can help investors and stakeholders make informed decisions.
Key Considerations in AI Integration
- Strategic Alignment: Does AI integration align with the company's long-term vision? In the cryptocurrency industry, this could mean automating trading algorithms or improving security protocols.
- Technological Readiness: Assess if the company has the infrastructure to support AI-driven products. In the blockchain space, this involves ensuring scalable and secure AI solutions.
- Regulatory Compliance: In crypto-related companies, AI must comply with regulations such as data privacy laws, anti-money laundering (AML), and know-your-customer (KYC) standards.
Steps to Evaluate AI in the Product Pipeline
- Analyze Current AI Projects: Review how AI is currently being used in the company’s offerings, such as AI-powered trading bots or decentralized finance (DeFi) platforms.
- Assess Market Potential: Evaluate the impact of AI on product competitiveness. Are AI features addressing a unique market need in the crypto industry?
- Track AI Partnerships: Look for collaborations with AI experts, research institutions, or tech firms. Strong partnerships can be a sign of robust AI capabilities.
"AI in cryptocurrency isn't just about improving operational efficiencies, it's about driving innovation in decentralized finance, security, and real-time market insights."
AI Integration in Cryptocurrency Product Development
AI Feature | Impact on Product |
---|---|
Automated Trading | Increases trading efficiency and decision-making speed |
Fraud Detection Algorithms | Enhances security and prevents fraudulent transactions |
Predictive Analytics | Forecasts market trends and optimizes investment strategies |
Risks to Consider When Investing in AI-Focused Businesses
Investing in AI-driven companies offers significant potential, but it's crucial to acknowledge the inherent risks. The AI sector is highly volatile and can be influenced by factors such as technological advancements, regulatory changes, and market sentiment. When considering AI businesses, particularly those that deal with cryptocurrencies and blockchain, investors need to weigh the risks carefully to avoid substantial losses.
As with any rapidly evolving industry, AI companies often face unforeseen challenges. These challenges can stem from competition, ethical concerns, and even the technology itself failing to deliver as promised. Understanding these potential pitfalls can help investors make more informed decisions and manage risks effectively.
Key Risks in AI-Focused Investments
- Regulatory Uncertainty: Governments are still developing frameworks for regulating AI and blockchain technologies. Any sudden changes in regulations can significantly impact AI businesses, especially those linked to cryptocurrencies.
- Technological Obsolescence: AI is a fast-moving field. Companies that fail to stay ahead of technological trends risk being outpaced by competitors, leading to declining stock prices.
- Market Volatility: Both AI and cryptocurrency markets are known for their high volatility. Price fluctuations can have a dramatic effect on investments, particularly in the short term.
- Data Privacy Concerns: AI systems rely heavily on data. Legal and ethical concerns around data privacy could lead to lawsuits or regulatory actions that harm a company’s reputation and market value.
Impact of Cryptocurrency on AI Investments
Cryptocurrency-based AI businesses face an added layer of risk due to the volatility and unpredictability of the crypto market. These companies often rely on blockchain technology, which can be susceptible to sudden regulatory crackdowns or market crashes.
"AI businesses that integrate cryptocurrency or blockchain are subject to double volatility, from both the AI industry and the unpredictable nature of digital currencies."
Summary of Risks
Risk Factor | Impact on Investment |
---|---|
Regulatory Changes | Can lead to sudden declines in stock value if new laws are unfavorable |
Technological Failures | Could reduce the company’s competitive advantage, impacting long-term profitability |
Market Volatility | Frequent price swings may cause instability in investment returns |
Data Privacy Issues | Can harm reputation and lead to legal complications, affecting financial performance |
Optimal Timing and Entry Strategies for AI Stock Investors
When navigating the stock market, particularly in the AI sector, knowing when to invest can be as important as choosing the right companies. Investors often look for signals that indicate favorable entry points–timing their moves in a way that maximizes potential returns. The AI industry, with its rapid growth and technological breakthroughs, presents unique opportunities, but also higher volatility. Understanding key moments, such as earnings reports, product launches, or advancements in AI technology, can provide crucial insights into optimal entry strategies.
Timing strategies revolve around market cycles, company fundamentals, and macroeconomic conditions. For AI stock investors, it's important to balance short-term fluctuations with long-term growth potential. Following these timing techniques can enhance the chances of purchasing stocks at favorable prices, setting up for significant returns as the sector continues to mature.
Key Entry Points for AI Stock Investors
- During Market Corrections: AI stocks, like any other sector, can face temporary downturns due to market-wide corrections. These periods often present buying opportunities for long-term investors.
- Post-Earnings Reports: After a company releases its earnings report, stock prices may drop or rise based on the results. Investors can capitalize on price movements after these announcements.
- When Technological Breakthroughs Occur: Significant advancements in AI can trigger a surge in stock prices. Keeping an eye on patent filings, research breakthroughs, or new partnerships can help investors identify ideal entry moments.
Effective Timing Methods
- Dollar-Cost Averaging: This strategy involves investing a fixed amount regularly, regardless of market conditions. It reduces the impact of short-term volatility and ensures consistent exposure to AI stocks.
- Technical Analysis: Investors can use chart patterns and technical indicators, such as moving averages and RSI, to gauge entry points. These tools help identify trends and potential reversal points in the market.
- News and Sentiment Analysis: AI stocks can be highly sensitive to news cycles. Monitoring announcements regarding new product launches, government regulations, or major investments can help investors predict stock price movements.
Important Considerations for Timing AI Investments
While AI stocks offer significant growth potential, it is important to recognize the risks associated with high volatility and market speculation. Careful analysis of trends and solid risk management are key to making the most of these investments.
Entry Point Table: AI Stock Timing Scenarios
Scenario | Recommended Action | Reasoning |
---|---|---|
Market Pullbacks | Buy on the dip | Corrective phases often provide discounts for stocks with strong fundamentals. |
Post-Earnings Announcements | Wait for price stabilization | Stock prices may react irrationally post-earnings, providing opportunities after stabilization. |
Tech Innovation News | Consider a position | Technological advancements can signal growth potential, driving stock prices upward. |